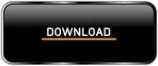
Proposes baseline pipeline for 2 stage object detection: generating region proposals and classifying them.But R-CNNs are very time-intense because it applies the CNN to about 2,000 warped selective search regions. This work combines works from classical CV and deep-learning for improving object detection. The performance supremacy of R-CNN compared to other methods come from the idea to perform the bottom-up style selective search also using CNNs to localize objects and the techniques used in fine-tuning the network on object detection data. Those cases are treated specially by providing a mixed label of the background and GT box class. When the box doesn’t significantly overlap with any GT box, or when the region has 0.5 IoU are considered to overlap wholly, the paper considers regions with 0.30.5 IoU to a ground truth (GT) box is considered that class and are trained to output the class of the GT box. Since the domain of the images is changed to images of warped windows, the classifier model is further trained on warped images and new labels. To reject overlapping region proposals in inference, where two or more bounding boxes are pointing to the same object, the authors propose a greedy algorithm that rejects a region if it has a high intersection-over-union (IoU) with another region that has a more confident prediction. The final stage is a fully connected layer, expressed as SVMs in the paper.The second stage is a fully convolutional neural network that computes features from each candidate region.Generate region proposals: the model must draw candidates of objects in the image, independent from the category.As described in the figure above, the overall pipeline is composed of three stages: The 2014 paper proposes the naive version of CNN based two-stage detection algorithm which is improved and accelerated in the following papers. The selection of papers on this post is mostly based on the survey. Therefore, it is important to understand all the main algorithms in two-stage detectors. These works are highly dependent on previous works and mostly build on the previous pipeline as a baseline. Such methods are known to be relatively slow, but very powerful, but recent progress such as sharing features improved 2 stage detectors to have similar computational cost with single-stage detectors. Deriving from the work of R-CNN, one model is used to extract regions of objects, and a second model is used to classify and further refine the localization of the object. One branch of object detectors is based on multi-stage models.